The integration of artificial intelligence (AI) into various medical fields has created many discussions about its potential to replace human roles. When it comes to medical coding in radiology, the question of “Will AI replace human medical coders?” often comes up, and at Reasint, we’ve heard this question many times. In this post, we’ll explore a few complexities and challenges of medical coding in the radiology field and look at what the ideal solution could be when thinking about human coders and AI.
The Context-Specific Nature of Medical Coding
Medical coding for radiology is not a one-size-fits-all process. It involves intricate conversations among coding managers, coders, and physicians, making it a highly context-specific activity. Certain straight-to-bill AI solutions, while efficient in some areas, can often lack the transparency, nuance, and contextual understanding necessary to navigate the dynamic coding environments common in modern healthcare settings. This is where human input is vital to keeping things on track.
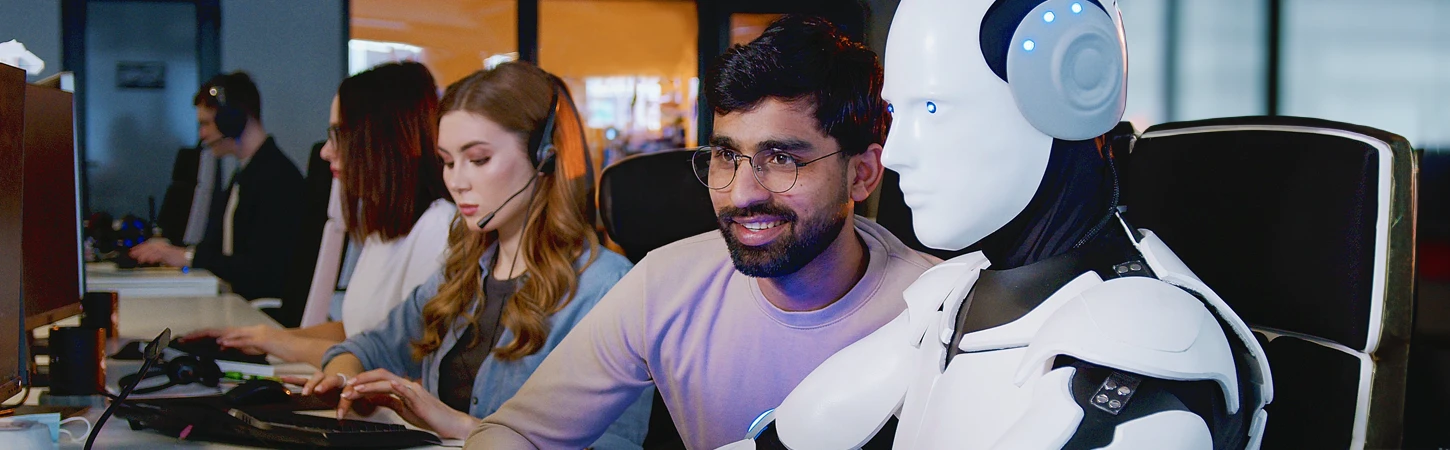
Constant Changes in Coding Regulations and Guidelines
The medical coding landscape experiences continuous updates, with regulations, authoritative guidance, codes, and definitions constantly evolving. In most instances, human coders can quickly adapt their behavior to these changes, while AI models often rely on training data that could be outdated by the time changes are put in place. The combination of human input and AI can help keep radiology coding systems from lagging behind in the rapidly changing coding world.
Handling Idiosyncratic Coding Activities
AI excels at recognizing patterns from large datasets, but radiology coding activities can often involve idiosyncratic cases, such as rare procedures or unique combinations of factors. Training data may not contain these unique cases, requiring human intervention to ensure accurate coding.
The diversity of coding scenarios demands the flexibility and judgment human coders bring to the table. Once AI is trained on these idiosyncratic scenarios, it can assist and optimize a human coder’s workflow, making it easier to achieve specific coding and billing goals.
Quick Turnarounds and Coding Errors
In the fast-paced production coding environment, quick turnarounds sometimes lead to erroneous or exaggerated coding behaviors. These are perhaps the biggest issues when it comes to human coding and AI assistance. Due to the sheer volume of reports they handle daily, human coders can overlook minute details, leading to either overcharge errors, financially impacting patients and third-party payers, or undercharges, resulting in financial losses for physicians.
Exaggerated coding also happens, often stemming from the limited opportunity to ask the physician about inconsistencies or missing details in documentation. In certain scenarios, coders may make assumptions about the services rendered, such as with screening mammograms.
Exaggerated Coding
While physicians routinely perform both mammograms and tomosynthesis procedures, the tomosynthesis may go undocumented. However, coders will still code for tomosynthesis, assuming it was conducted. This assumption is known as exaggerated coding, even though it is considered accurate when acknowledging the medical group or clinic is expected to perform tomosynthesis.
AI training models can magnify these issues, especially since the accepted standard for AI direct-to-bill percentage in the industry is 85%. AI coding firms actively market and commit to delivering this benchmark, facing significant expectations to uphold it in practical coding scenarios.
However, when AI is trained using data from human coders, whose records are often rife with errors and exaggerated coding, the AI tends to adopt and replicate these patterns. In many cases, the AI’s adoption of these tendencies exceeds the scope of human coders, resulting in an amplified error rate in coding.
People + AI: The Optimal Solution
Rather than viewing AI as a total replacement for human medical coders in radiology, it’s more effective to recognize the complementary strengths of both. While AI can efficiently handle common, recurring problems, human coders excel in addressing the unique challenges posed by complex and evolving coding scenarios. This beneficial synergy between humans and AI in medical coding ensures a comprehensive and accurate approach.
Looking Ahead
In the dynamic and context-specific field of radiology medical coding, collaboration between human expertise and AI capabilities is the optimal solution and answer to the question of whether or not AI will replace human medical coders. Embracing a people + AI approach allows for the efficient handling of routine tasks by AI, while preserving the critical thinking and adaptability of human coders in navigating the complexities and challenges of medical coding in radiology.
At Reasint, we’re dedicated to helping human coders discover the future of medical coding intelligence and how AI can help you work better, faster, and more efficiently. Schedule a demo now to learn more!